- Lab
- Data
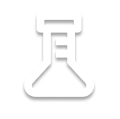
Integrate Databases with Apache Airflow
This lab introduces you to the fundamentals of integrating databases with Apache Airflow, a key technique for automating and managing data workflows across distributed systems. You will explore core Airflow functionalities, including configuring database connections, executing SQL queries, and handling data processing tasks using Airflow’s built-in operators. Throughout this lab, you will gain hands-on experience in establishing a connection between Airflow and MySQL, executing SQL queries using MySqlOperator, and processing data within Python tasks. By applying these techniques, you will develop a deeper understanding of how Apache Airflow facilitates database integration, improves workflow orchestration, and enhances data pipeline efficiency. This lab is designed for data engineers, analysts, and developers looking to refine their skills in managing and automating database interactions using Apache Airflow. By the end of this lab, you will have the expertise to design and implement reliable database workflows that seamlessly integrate with Apache Airflow.
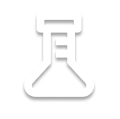
Path Info
Table of Contents
-
Challenge
Introduction Integrate Databases with Apache Airflow
Introduction to Integrating Databases with Apache Airflow
In this lab, you will build an automated data pipeline using Apache Airflow to extract, transform, and store employee data using SQLite. Airflow is a powerful workflow orchestration tool that enables scheduling, managing dependencies, and automating data processes efficiently.
By working with employee records, you will learn how to extract data from SQLite, apply transformations, store processed records, and execute SQL operations through Airflow DAGs.
🟦 Why It Matters
Understanding how to integrate databases with Apache Airflow is essential for automating ETL (Extract, Transform, Load) workflows. This lab will help you:
- Automate data extraction from an SQLite database using Airflow operators.
- Transform extracted data by applying salary adjustments and categorizing employees.
- Store processed records in a structured relational database table for further use.
- Execute SQL operations within Airflow DAGs, ensuring seamless database interactions.
- Trigger and monitor DAG execution, verifying that each step of the workflow is successfully executed.
🔍 Key Concepts
Extracting Data with Airflow
- Purpose: Retrieve structured employee records from SQLite using Airflow’s
SqliteHook
. - Implementation: Execute SQL queries within an Airflow DAG to extract data into a Pandas DataFrame.
- Use Case: Extracting employee records and storing them for further processing.
Transforming and Processing Data
- Purpose: Modify extracted data by adjusting salary values and categorizing experience levels.
- Implementation: Use Pandas transformations to update salary values and classify employees.
- Use Case: Standardizing salary figures and categorizing employees as Junior or Senior based on experience.
Storing and Managing Data in a Relational Database
- Purpose: Save processed records in a structured SQLite table for easy retrieval.
- Implementation: Use Airflow DAGs to create tables and insert transformed records into the database.
- Use Case: Storing processed employee data with categorized experience levels for analysis.
Automating Workflow Execution with Airflow DAGs
- Purpose: Orchestrate and automate the data pipeline using scheduled tasks.
- Implementation: Define PythonOperator tasks to execute each step of the process within an Airflow DAG.
- Use Case: Automating the entire process from data extraction to database storage.
🟩 Learning Objectives
By the end of this lab, you will:
- Extract data from an SQLite database using Airflow’s
SqliteHook
. - Transform extracted data by applying modifications to salary values and experience categories.
- Save transformed data to a new database table for structured storage.
- Define and execute an Airflow DAG to automate the ETL process.
- Trigger and verify DAG execution, ensuring that each step runs successfully.
Now that you have an overview of database integration with Apache Airflow, let's start building the workflow! Click on the Next Step arrow to begin 🚀.
-
Challenge
Extract Data from Database
Step 1: Extracting and Storing Employee Data
In this step, you will extract employee records from an SQLite database and store them in a structured format for further analysis. This process involves establishing a connection to the database, retrieving employee data, and saving it as a CSV file.
By the end of this step, you will have a fully automated Airflow task that connects to SQLite, extracts employee records, and writes them to a CSV file.
🟦 Why It Matters:
- Database extraction is the foundation of any data pipeline, ensuring access to structured and reliable information.
- Persisting extracted data in a CSV file allows for easy analysis, sharing, and integration with other tools.
- Defining tasks in an Airflow DAG enables automation and scheduled execution of data extraction processes.
In Airflow, you will:
- Establish a connection to an SQLite database, ensuring Airflow can retrieve employee records.
- Extract data from the
employees
table and store it in a Pandas DataFrame. - Save the extracted employee data as a CSV file, making it available for further processing.
- Register the extraction process as an Airflow task to enable automation and scheduled execution.
Dataset Schema
The
employees
table contains structured information about company employees, including their department assignments, salary details, and experience.Employees Table Schema
| Column Name | Data Type | Description | |-----------------|----------|-------------| |
employee_id
| Integer | Unique identifier for each employee | |name
| String | Full name of the employee | |age
| Integer | Employee’s age | |department
| String | Department name the employee belongs to | |salary
| Float | Employee’s salary | |experience
| Integer | Years of experience in the company |This dataset allows structured workforce analysis, enabling insights into department-wise employee distribution, salary trends, and experience levels.
By the end of this step, you will have a fully automated data extraction workflow that ensures employee data is consistently available for analysis and reporting.
-
Challenge
Process Extracted Data
Step 2: Processing and Transforming Employee Data
In this step, you will process and transform the extracted employee data to enhance its usability for analysis. This involves loading the extracted data from a CSV file, applying transformations to adjust salary values, categorizing employees based on experience, and saving the processed data for further use.
By the end of this step, you will have a clean and structured dataset with standardized salary values and categorized experience levels, ready for storage or additional analysis.
🟦 Why It Matters:
- Data transformation ensures consistency and prepares raw data for meaningful insights.
- Adjusting salary values allows for financial modeling and workforce compensation analysis.
- Classifying employees by experience helps in understanding workforce distribution and career progression.
- Saving the processed data in a structured format ensures its availability for future reporting and analysis.
In Airflow, you will:
- Load extracted employee data from a CSV file into a Pandas DataFrame.
- Apply transformations to increase salaries by 10%, ensuring consistent formatting.
- Categorize employees as Junior or Senior based on experience levels.
- Save the transformed dataset for future analysis.
- Trigger the Airflow DAG to execute the processing workflow and verify that the data has been correctly transformed.
By the end of this step, you will have a fully processed and structured dataset, making it easier to analyze workforce trends, compare salaries, and categorize employees based on experience.
Observation: Triggering the DAG and Verifying Processed Data
Now that the extracted employee data has been processed, you can manually trigger the Airflow DAG to execute the transformations and verify the output.
This process is very similar to Task 1.4, where you triggered the DAG to extract and save raw employee data. However, in this observation, you will verify that the transformations—salary adjustments and experience categorization—were applied correctly.
🛠 Steps to Execute and Verify
-
Trigger the DAG manually from the terminal:
airflow dags trigger extract_employee_data
-
Verify the processed CSV file:
- Run this command to check the contents of the transformed data:
cat /tmp/processed_employees.csv
- If the DAG executed successfully, this command will display the first 10 rows of the processed employee data.
- Run this command to check the contents of the transformed data:
🔍 Expected Outcome
After running
cat /tmp/processed_employees.csv
, you should see employee details with updated salary and categorized experience levels:employee_id,name,age,department,salary,experience,experience_category 1,Alice Johnson,34,HR,71500.0,10,Senior 2,Bob Smith,28,Engineering,93500.0,5,Junior 3,Charlie Brown,45,Finance,101200.0,18,Senior 4,Diana Green,29,Marketing,82500.0,7,Junior 5,Ethan White,40,HR,66000.0,12,Senior 6,Fiona Black,35,Engineering,106700.0,9,Junior 7,George Clark,50,Finance,121000.0,25,Senior 8,Hannah Blue,31,Marketing,79200.0,6,Junior 9,Ian Gray,42,Engineering,112200.0,20,Senior 10,Jane Miller,37,HR,77000.0,14,Senior
If the file is empty or the transformations are incorrect, ensure that:
- The DAG was triggered successfully.
- The processing task executed without errors.
- The
processed_employees.csv
file was correctly updated.
Once verified, your transformed employee data is now ready for further processing!
-
Challenge
Load Processed Data into Database
Step 3: Storing Processed Employee Data in the Database
In this step, you will store the transformed employee data into a structured database table. This ensures that the processed data, including standardized experience classifications, is available for querying and further analysis. You will define a table for storing the processed data, insert the transformed records, and configure the execution order of the Airflow DAG to ensure a seamless workflow.
By the end of this step, you will have a fully automated data pipeline that extracts, processes, and stores employee data in a structured format.
🟦 Why It Matters:
- Storing transformed data in a database ensures it is accessible for reporting and analysis.
- Creating a dedicated table for processed data allows structured storage and easier querying.
- Ensuring the correct execution order in Airflow maintains data integrity and prevents issues in workflow execution.
- Automating data insertion into the database eliminates manual effort and ensures up-to-date records.
In Airflow, you will:
- Create a new table for processed employee data in the database.
- Insert only relevant fields (name, experience, experience category) from the transformed dataset.
- Define the correct execution order so that data processing happens before insertion.
- Trigger the Airflow DAG to execute the database storage workflow and verify that the data is correctly stored.
By the end of this step, you will have a fully structured and automated workflow that extracts, processes, and stores employee data efficiently, ensuring it is available for future analysis.
Observation: Triggering the DAG and Verifying Stored Processed Data
Now that the transformed employee data has been successfully stored in the database, you can manually trigger the Airflow DAG to execute the final step and verify that the records have been inserted correctly.
This process is very similar to previous verification steps, where you checked the extracted and processed CSV files. However, in this observation, you will validate that the transformed data—including experience categorization—has been inserted into the database.
🛠 Steps to Execute and Verify
-
Trigger the DAG manually from the terminal:
airflow dags trigger extract_employee_data
-
Verify the stored processed data in SQLite:
-
First, open an SQLite shell and connect to the Airflow database:
sqlite3 ~/airflow/airflow.db
-
Retrieve the first 10 rows of inserted processed employee data:
SELECT * FROM processed_employees LIMIT 10;
-
🔍 Expected Outcome
After running
SELECT * FROM processed_employees LIMIT 10;
, you should see employee records with only name, experience, and experience category:1|Alice Johnson|10|Senior 2|Bob Smith|5|Junior 3|Charlie Brown|18|Senior 4|Diana Green|7|Junior 5|Ethan White|12|Senior 6|Fiona Black|9|Junior 7|George Clark|25|Senior 8|Hannah Blue|6|Junior 9|Ian Gray|20|Senior 10|Jane Miller|14|Senior
If the table is empty or the records are missing, ensure that:
- The DAG was triggered successfully.
- The table creation task was executed.
- The data insertion task ran without errors.
Once verified, your transformed employee data is now successfully stored and ready for further use!
--- # 🎉 Congratulations on Completing the Lab!
You have successfully completed the Integrate Databases with Apache Airflow lab. Throughout this lab, you built an end-to-end data pipeline using Apache Airflow, SQLite, and Pandas to extract, process, and store employee data.
In this lab, you learned:
- Extract employee records from an SQLite database using
SqliteHook
. - Store extracted data in a CSV file to ensure data persistence.
- Apply transformations by increasing salaries and categorizing employees by experience.
- Save processed data into a structured format for further analysis.
- Create and manage an SQLite table for processed employee records.
- Insert transformed data into a relational database for storage and reporting.
- Trigger Airflow DAGs to automate the pipeline and verify processed data.
Key Takeaways
Automating Data Extraction
- Using Airflow’s
SqliteHook
, you connected to SQLite and extracted structured employee data. - Extracted data was saved into a CSV file, ensuring it was available for further transformation.
Transforming and Processing Data
- Salary values were increased by 10%, ensuring consistent salary adjustments.
- Employees were categorized as Junior or Senior based on experience levels.
- Transformed data was stored in a new CSV file for easy verification.
Storing Processed Data in a Relational Database
- A new SQLite table (
processed_employees
) was created to store transformed data. - The table was structured with only name, experience, and experience category to keep it concise.
- Data was inserted into SQLite from the processed CSV file, ensuring persistence.
Executing and Validating the DAG
- The Airflow DAG was manually triggered to execute each step of the pipeline.
- The processed data was verified in both CSV format and SQLite database using SQL queries.
Congratulations again on successfully completing the lab! 🎯
What's a lab?
Hands-on Labs are real environments created by industry experts to help you learn. These environments help you gain knowledge and experience, practice without compromising your system, test without risk, destroy without fear, and let you learn from your mistakes. Hands-on Labs: practice your skills before delivering in the real world.
Provided environment for hands-on practice
We will provide the credentials and environment necessary for you to practice right within your browser.
Guided walkthrough
Follow along with the author’s guided walkthrough and build something new in your provided environment!
Did you know?
On average, you retain 75% more of your learning if you get time for practice.